- About
- Events
- Societies and Chapters
- AP/ED/MTT
- AP / VTS / EMC Joint Chapter
- Broadcast Technology Chapter
- Circuits and Systems Chapter
- Computer Society / Princeton ACM
- Consumer Electronics and Communications Chapter
- Education Society
- Engineering in Medicine and Biology Chapter
- PELS & IAS
- Photonics Society
- Power and Energy Society
- Robotics and Automation Society
- Signal Processing Chapter
- Solid-State Circuits Society
- Affinity Groups
- Archives
- Gallery
- Picnic July 11, 2024
- Awards 2024
- David Soll
- Holiday Party 2023
- Holiday Party 2022
- IEEE PCJS 75th Anniversary Picnic, 8/25/22
- Holiday Party Dec 2021
- PCJS Picnic July 2021
- HolidayPartyDec2019
- Awards 2019
- Photo_NetworkAuto
- Photo_ExComFeb2019
- HolidayParty2018
- Sarnoff2018
- Awards2018
- ExComSept2018
- History Region / IEEE
- Join IEEE
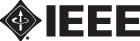