GEOMETRIC LEARNING FOR SHAPE ANALYSIS FROM BIOIMAGING DATA – 19 April @ 6PM
IEEE CENTRAL COAST EVENT – 19 APRIL – PROF. NINA MIOLANE PRESENTS “GEOMETRIC LEARNING FOR SHAPE ANALYSIS FROM BIOIMAGING DATA”
LOCATION – Rusty’s Pizza 5934 Calle Real, Goleta, CA 93117
6:00 PM – Complimentary Pizza, Salad, Beverage
6:25 PM – Central Coast Status
6:30 PM – Dr. Miolane Presents
Greetings, You and guest(s) are invited to join us at Rusty’s Pizza on April 19th at 6PM for an exciting talk by Dr. Nina Miolane (UCSB ECE) in which she presents studies showing the theory and applications of Geometric Statistics to the analysis of biomedical shape data.
Link to Register yourself and guests: https://events.vtools.ieee.org/event/register/355459
GEOMETRIC LEARNING FOR SHAPE ANALYSIS FROM BIOIMAGING DATA
Advances in bioimaging techniques have enabled us to access the 3D shapes of a variety of structures: organs, cells, proteins. Since biological shapes are related to physiological functions, statistical analyses in biomedical research are poised to incorporate more shape data. This leads to the question: How do we define quantitative descriptions of shape variability from images?
Mathematically, landmarks’ shapes, curve shapes, surface shapes, or shapes of objects in images are data that belong to non-Euclidean spaces, for example to Lie groups or quotient spaces. In this context, we introduce “Geometric statistics,” a statistical theory on non-Euclidean spaces. We present studies showing the theory and applications of Geometric Statistics to the analysis of biomedical shape data.

Dr. Nina Miolane received her MS in Mathematics from Ecole Polytechnique (France) & Imperial College (UK), and her PhD in Computer Science from INRIA (France) in collaboration with Stanford University. She was an instructor in the French Army for a year and was decorated for succeeding in a commando training program. After her studies, Nina spent two years at Stanford University in Statistics as a postdoctoral fellow, and worked as a deep learning software engineer in the Silicon Valley.
At UCSB, Nina directs the BioShape Lab, whose goal is to explore the “geometries of life.” Her research investigates how the shapes of proteins, cells, and organs relate to their biological functions, how abnormal shape changes correlate with pathologies, and how these findings can help design new automatic diagnosis tools. Her team also co-develops the open-source Geomstats library, a software that provides methods at the intersection of geometry and machine learning, to compute with geometric data such as biological shape data.
Nina is a co-author of the book Riemannian Geometric Statistics For Medical Imaging and a co-inventor on patents in computational medicine. Her research funding includes an NIH R01 grant on Biological and Mathematical Science and the NSF SCALE MoDL grant on Mathematical and Scientific Foundations of Deep Learning. Nina was the recipient of the L’Oreal-Unesco for Women in Science Award and is involved in outreach initiatives in France and in the US. In her free time, Nina adventures in the golden state’s outdoors, hiking in the mountains, riding motorcycles along the coast, or piloting single-engine airplanes.
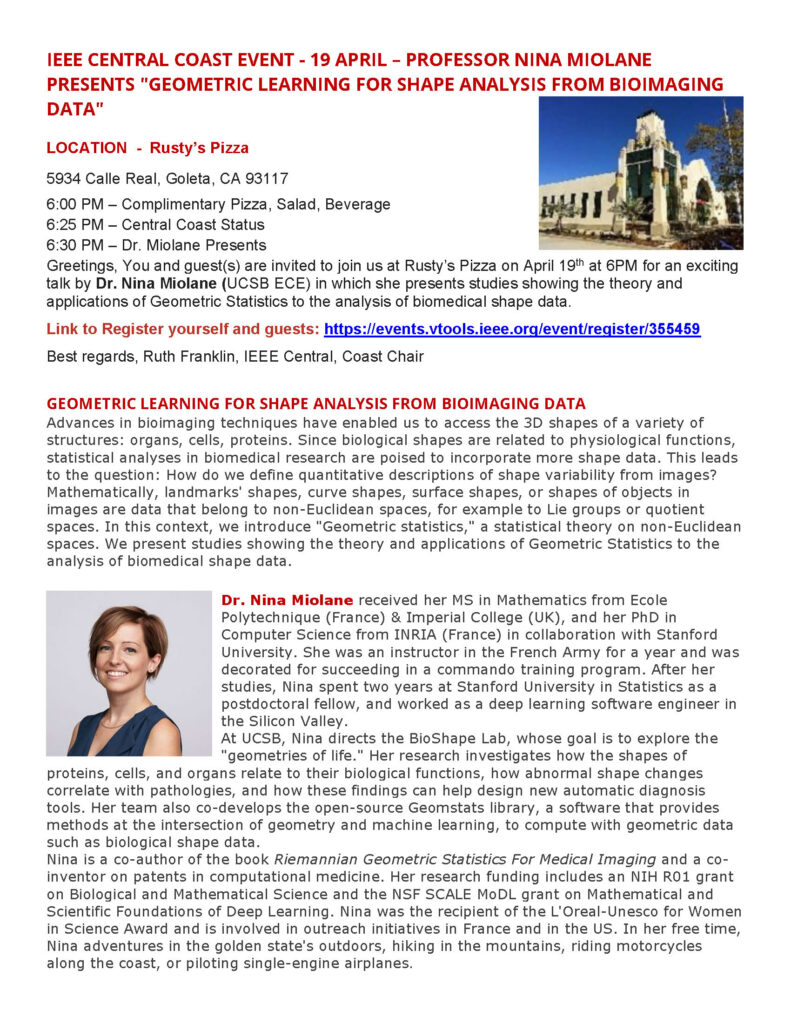